S2-Episode 1: Real or fake? The buzz about synthetic data
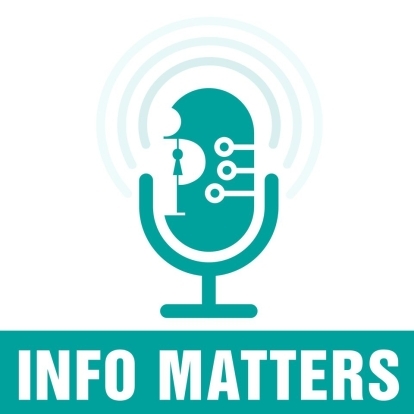
New artificial intelligence technologies now make it possible to create fake versions of personal information that look and feel a lot like the real thing but are different enough to protect individual privacy. In this episode, Commissioner Kosseim speaks with Dr. Khaled El Emam about how synthetic datasets are made from real patient data and how they can be used to advance important health research while minimizing privacy risks.
Notes
Dr. Khaled El Emam is a Canada Research Chair in Medical Artificial Intelligence and a professor in the University of Ottawa’s Faculty of Medicine. He is Senior VP and General Manager of Replica Analytics, an Aetion company.
- Developing privacy enhancing technologies to enable access to health data to support research [3:46]
- What is synthetic data? [5:36]
- Anonymous versions of real clinical data sets [8:36]
- Testing synthetic data to determine privacy risks [9:02]
- Examples of real world use cases in Canada [10:06]
- Re-identification attacks and loss of trust in traditional methods [12:31]
- Comparing statistical properties of synthetic data to the real thing [14:15]
- Is synthetic data really just like the real thing? [14:21]
- How synthetic data is made using artificial intelligence technologies [15:05]
- Use of synthetic data for research is expected to accelerate, increasing the need for practical regulatory guidance [16:47]
- Reducing uncertainty to fuel innovation [19:30]
- The need for clear rules around consent and thresholds for identifiable versus unidentifiable data [23:13]
Resources:
- The Fourth Industrial Revolution: What it means, how to respond (article by Klaus Schwab)
- Even better than the real thing: Simulated, anonymized data could be key to health-care innovations (University of Alberta research project)
- Top 10 strategic predictions for 2022 and beyond (Gartner)
- Trust in digital health: IPC strategic priorities 2021-2025 (IPC strategic plan)
- IPC Comments on the Ontario Government’s Consultation on Ontario’s Trustworthy Artificial Intelligence (AI) Framework (IPC Submission, June 2021)
- De-identification guidelines for structured data (IPC guidance)
Info Matters is a podcast about people, privacy, and access to information hosted by Patricia Kosseim, Information and Privacy Commissioner of Ontario. We dive into conversations with people from all walks of life and hear stories about the access and privacy issues that matter most to them.
If you enjoyed the podcast, leave us a rating or a review.
Have an access to information or privacy topic you want to learn more about? Interested in being a guest on the show? Send us a tweet @IPCinfoprivacy or email us at @email.
Transcripts
Patricia Kosseim:
Hello, I’m Patricia Kosseim, Ontario’s Information and Privacy Commissioner, and you’re listening to Info Matters, a podcast about people, privacy, and access to information. We dive into conversations with people from all walks of life and hear real stories about the access and privacy issues that matter most to them.
Hello, listeners, and thanks for tuning in. Klaus Schwab, the Founder and Executive Chairman of the World Economic Forum once said, “We stand on the brink of a technological revolution that will fundamentally alter the way we live, work, and relate to one another. The transformation will be unlike anything humankind has experienced before.” Well, we are now into what he’s dubbed the fourth Industrial Revolution that builds on the digital transformations of the early two 2000s, with the introduction of things like artificial intelligence, advanced robotics, and embedded connectivity that are literally blurring the lines between our physical, digital, and biological worlds. All of this of course is driven by an ocean of data out there generated by individuals, organizations, businesses, and machines made possible due to massive storage and processing power.
And although we may feel completely awash with more data than we can handle, sometimes we now have the technology through AI and machine learning to help us cut through all the noise and make sense of it all. We now have ways to gain meaningful insights and make data-driven decisions to solve some of society’s most pressing problems in areas such as health, education, and the environment, and help us realize broader societal benefits. Some call this data for good.
As healthcare costs continue to rise and pressures on the health system continue to escalate, aggravated by this unprecedented and unrelenting pandemic, decision makers are faced with tough choices about how to make best use of the limited resources available. Some believe the key lies in analyzing and gaining insights into health data, combined with other sociodemographic and economic data to help us get a more complete picture and understanding of medical conditions, leverage innovations to develop new treatments, and manage resources in a way that lowers costs and improves overall quality of life. But how can we expand access to useful data without compromising the privacy of individuals? Is there a way to create a stand-in for real data that can deliver similar results, a way of replicating the data so to speak, or at least its statistical patterns in non-identifiable ways? Well, it turns out there is. It’s called synthetic data. And that’s what this episode is all about.
My guess for today is Dr. Khaled El Emam. Dr. El Emam is an electrical and electronic engineer by background and currently holds the Canada Research Chair in Medical Artificial Intelligence at the University of Ottawa. He’s also the CEO of Replica Analytics, a company that develops software for generating synthetic data that protects privacy of individuals while maintaining the statistical properties of real data. Khaled, welcome to the show.
Khaled El Emam:
Thank you very much for having me.
PK:
I’ve known you for many years, and I know you’ve dedicated almost your entire career to developing privacy-enhancing technologies. In fact, you founded a couple of successful startup companies that offer these kinds of services. What led you into research and development of new techniques and approaches for anonymizing data?
KE:
We can go back to the early days. When I first started working on this topic, I was involved with health research. So really the genesis was enabling access to data for health research. A lot of innovations for drug development and for understanding the progression of disease relies on data and relies on the analysis of data that has already been collected. Data collected at clinics, data collected at hospitals and so on. And access to this data has historically been very challenging due to privacy concerns, not because the regulations make it difficult, just because the interpretation of these regulations is sometimes overly conservative and to really implement the regulations requires some advanced technology. So I started working on developing some of these technologies that would enable access to health data, to support research, to support drug discovery, adverse events from using drugs or vaccines and so on, and to make this data accessible and available to researchers as efficiently as possible, but also maintain the quality of that data.
So the class of technologies here is called privacy-enhancing technologies. And what they effectively do is create anonymous versions from the real data. So you take a real data set, a real clinical data set, and you would create an anonymous version that is not linked to people. My initial adventures in this space was to develop some kind of risk-based anonymization technologies. And over time we improved on those and developed more sophisticated methods, which we’ll talk about more later on.
PK:
One of those is synthetic data. I’d like to ask you if you can tell us, first of all, what is synthetic data and share some real-life examples of its use with our listeners?
KE:
Yes, absolutely. Synthetic data is essentially fake data. I’m sure a lot of people have seen “deep fakes,“ which are the fake images that look very realistic. They are images that are generated from AI models. So the basic idea is you get a large bank of images of real people that you train some AI models to understand what real people look like, what are the characteristics of real faces, et cetera. And then once you’ve trained those AI models, you can use them to generate new images, new faces. And the technology has advanced so much that these new images, these deep fakes basically look very real.
Let’s take an example of a maternal and infant health database. This is the type of data that’s collected during pregnancy and after an infant is born. And there are a lot of pieces of information that can be collected around the health of the mother, around smoking status, around gestational age, birth weight, and generally the health of the infant. And so if you take that data set and create a synthetic derivative of it, you maintain the same proportions in the synthetic data – women who smoke and don’t smoke, for example, the proportion of male and female infants, the distributions of gestational age and birth weight. So all of these characteristics are maintained in the data set. And imagine you’re doing this across maybe hundreds of different attributes that maybe in this database. But it’s not just these individual characteristics. Also, the patterns of the data where the relationships in the data are maintained. And there may be many, many relationships among these hundreds of attributes. For example, if there’s a relationship between smoking status and birth weight in the real data, then that relationship would also be maintained in this synthetic data.
No record in the synthetic data will have or could have exactly the same values on all the attributes as a record in the real data, but across all of them, for example, that pattern between smoking and birth weight would still be maintained. So you would still draw the same conclusions about this relationship by analyzing or by examining the synthetic data as if you had the original data. So at the individual level, the values are not the same, but an aggregate across all of them, across all the births in this data set, the patterns are the same.
PK:
If I were a woman in your study in the original data set, for instance, and you were to replicate the values in a synthetic data, you would have general attributes that would look the same, but no individual record in the synthetic data would look exactly like me with all the exact same attributes and traits that make up who I was or who I am in the original data set.
KE:
Exactly. But also this can be tested. There are ways to evaluate synthetic data and determine what are the privacy risks in that synthetic data. So to what extent does the synthetic data replicate exactly the patterns in the real data or patterns about real people? So you can test that. And if the risk is high, you can go back and redo it and get a new synthetic data set that reduces the risk. So there are ways to test this. You don’t have to take it at face value. You can do it, evaluate it and make sure that these risks are small because they’re not real data. They’re generated from these AI models, they have a lot of privacy protective characteristics. So we achieve our objective of creating anonymous information, but that’s still very useful because it maintains the patterns. There’s no data, it is realistic data. That’s what synthetic data is.
In terms of uses, I mean, there are a few public examples that I can talk about. One of them is some work we did in Alberta with the University of Alberta and health cities there and Edmonton, where we took health system data sets. The intention was to make that type of data available to researchers at the university and we wanted to see whether synthetic data could solve the problem. And it did. We created synthetic versions of quite complex health system data sets. And when the researchers at the University of Alberta analyzed the data, they got the same results with the synthetic data that they did with the original data.
So it can work quite well in practice. Statistics Canada has used synthetic data to support hackathons, for example. And the Canadian Institute for Health Information also gave a presentation recently where they’re talking about their interest in using synthetic data for… They hold a lot of health data. So synthetic data as a way to enable access to some of their data holdings. The RAMQ and Quebec also has been looking and exploring the uses of synthetic data to enable access and data sharing. So there are various initiatives across the country that are using and applying synthetic data as a means to solve the data access problem.
And then another, I think important use case going back to health is around rare diseases. Rare diseases as the name implies are rare. So you don’t have enough data for analysis. And so synthetic data generation techniques can create virtual patients. So again, you train these AI models to learn the properties and the patterns in those patients’ data. And then once you’ve learned those patterns, you can then create or synthesize virtual patients, and that you’d have more data to use for analysis and for research to discover new patterns or test hypotheses with that data. I would say these are some of the main use cases today, that have emerged around applications of synthetic data generation techniques.
PK:
So why move towards the use of synthetic data instead of using real data that’s been de-identified? What are some of the benefits of synthetic data?
KE:
Yeah, the de-identification methods that have been in use for say 10 to 20 years or so, they have historically worked quite well, but in practice, there’ve also been these re-identification attacks. And because of this, the narrative around traditional de-identification methods has become more negative over time, which results in an erosion of trust, erosion of trust by regulators, erosion of trust by the public. That’s one challenge that we’re facing.
And then another challenge is traditional de-identification methods require quite a bit of expertise and skills, and those skills are quite difficult to find. They require a lot of training and a lot of technical knowledge to do well. So these two challenges, the negative narrative and the lack of skills have made it difficult for organizations to use and share and really fully utilize their data sets. We needed something new.
So synthetic data solves some of these problems. It doesn’t require as much skill because it’s a largely automated process. That’s just a very practical benefit. And then it doesn’t have a negative narrative around it. So there’s more acceptance of it as the way forward to enable the sharing of data. And the results so far are encouraging in the sense that it is privacy protective and it does a good job in ensuring that the privacy risks are quite small. This is why synthetic data at least today is attractive and my hypothesis is that it’s the future of data sharing moving forward.
PK:
How well does synthetic data compare to the real thing in terms of statistical properties?
KE:
It’s not going to be exactly as the real thing, because if it’s exactly same as the real thing, then you’re essentially replicating or copying the real data. So there’ll always be some differences and you should always expect to see some differences. The objective though, is that the synthetic data captures the patterns in the original data, so that the analysis that’s done with the synthetic data results in the same conclusions as the analysis that you would do on the real data. And the evidence so far has been encouraging on that front.
PK:
Maybe for our non-technical listeners, can you explain in easy terms, how do you actually make synthetic data?
KE:
It uses AI and artificial intelligence techniques kind of broadly defined, and you start with a real data set. So let’s take a concrete example. Let’s say it’s a hospital database, a hospital data set that you want to create a synthetic version of. So you get the hospital data set and you train an AI model using that hospital data set. And then once you have a trained model, you essentially make that AI model generate new data based on all the patterns that it has learned. And this new data will look like the original data, because it’s following the same patterns that were learned by the AI model. So this works really well if the AI model is good, if it really captured the patterns in the original data. And there have been enough advances in this area that these AI models have become really good at capturing very subtle and complex patterns in the original data, and it’s continuously improving.
So when you generate the synthetic data this way, it looks like the real data. It has the same patterns, the same distribution, same statistical properties as the original data. And when you use it to do analysis or to visualize patterns, you will see very similar patterns and draw the same conclusions as if you had the original data.
PK:
This is cutting-edge stuff. This is all new area of study and exploration. How ready are we for synthetic data from a legal and policy perspective? Do we have the right frameworks in place to permit its use for the good purposes you laid out?
KE:
Well, let me step back a little bit and just talk about adoption of synthetic data in general. I mean, we’re seeing increased interest for sure. And then some of the big analyst companies like Gartner and Forrester have been predicting that most data that’s used for artificial intelligence purposes will be using synthetic data in the next few years. Just because real data is hard to get access to, that for modern applications of AI, large amounts of data are needed. So their prediction is that synthetic data will solve that problem. Their predictions for the adoption of synthetic data show quite an aggressive adoption curve over the next five years. And then Forrester identified synthetic data generation as one of the top AI technologies in the future. For all of these reasons, the adoption curve I think is accelerating quite fast.
In terms of what should be done, I mean, given the rapid acceleration and adoption, there’s a real need for regulatory guidance. Whether, for example, synthetic data should be treated the same way as traditional anonymized or identified data, or should it be treated differently because it’s not real data. It comes from models rather than derived directly from real data. So there are a couple of considerations here and having some concrete guidance on how synthetic data should be treated. There’s some movement now to regulate anonymized data to some extent. Should synthetic data be regulated, or because it’s not real data, it should be treated differently? These are the questions that are coming up, and it’ll be very beneficial to support the adoption of synthetic data and to ensure that it’s used responsibly and users and technology providers for synthetic data are putting in place the right controls and the right mechanisms to manage the risks. Regulatory guidance would be very helpful at this point. This is the time to intervene and make a difference in the trajectory of how this technology will evolve.
PK:
Actually, that’s a good segue for my next question, which is how can an office like mine or other data protection regulators help advance the debate and the discussion on technologies such as this, to help resolve some of the de-identification challenges we’ve seen and the promises it holds for many socially beneficial uses of data?
KE:
I mean, your office has produced de-identification guidance which was I think a fantastic document because it provided very operational and concrete guidance on de-identification a few years ago. And I think something like expanding such guidance to synthetic data generation or other types of privacy-enhancing technologies would be very beneficial. My observation is that uncertainty creates paralysis. And whenever there’s uncertainty about the use of a particular technology or how a particular technology, especially a new one is going to be regulated, many organizations just wait to see what happens. They are less willing to take a risk by using a new technology when the regulatory regime is unclear. So increasing our uncertainty, I think is always beneficial in terms of providing application guidance that’s as operational as possible, that’s even better to reduce this uncertainty. And I think that would have a huge impact on responsible uses of the technology, but also to support adoption.
PK:
One of our strategic priority areas that we identified and that we’re working on actively is Trust in Digital Health. And you mentioned something important about how important trust is to the possibility of using and sharing data for these purposes. In your view, how does synthetic data contribute to enhancing or building trust in digital health systems?
KE:
A number of things can be done. Synthetic data itself, just because it has a low risk of identifying individuals, it’s a good way to share data and provide access to data in a way that respects people’s privacy or patient’s privacy. So it allows the data to be used for beneficial purposes that are really quite important for society. There’s still many issues to be solved, but I think the pandemic really highlighted the importance of data access. So synthetic data allows us to do that in a way that’s responsible and protects the rights of the individuals.
But the others side of this is how do we know that the data that we are producing is used in acceptable ways, unsurprising ways? You’re not going to build models from the data that will discriminate against certain individuals, or that will make creepy decisions or surprising decisions about individuals. And I think this is an important issue, and it’s more of an ethics issue where there has to be an ethics overlay over how data, non-identifiable data, synthetic data is used and what kind of decisions are made from that data. I think these two things will go a long way to building that trust or ensuring trust and information sharing.
PK:
As you know, there’s a whole raft of legislative reforms underway here in Canada, among the provinces, internationally. And this new generation of privacy laws is evolving at a very rapid pace. What would you see as some of the concrete amendments that you think would be needed in a modern privacy law to allow these types of new technologies like synthetic data to really lift off and take flight for solving some of the world’s important social problems?
KE:
Yeah. I think at a high level, there are two big things. One is reducing uncertainty, and the other one is incentives. Let me give some examples. Two big things that often contribute to this uncertainty are whether additional individual consent is needed to create non-identifiable data such as synthetic data. And some regulations such as the Ontario’s Health Law are very clear in that the consent is not required, but other statutes across the country are ambiguous about this. And therefore, the community at large, organizations and the public and private sector have to read in what they should do with respect to consent. Now, a strong argument can be made that methods to create non-identifiable data so that data can be used for analysis and research and so on, is a good privacy protective measure.
Non-identifiable data is better for the individuals. It’s a good way to protect their rights. And also it can enable beneficial uses of data. And so an argument can be made that the act of creating non-identifiable data such as synthetic data does not or should not require additional consent. So by being explicit about this, that reduces uncertainty and it enables organizations to apply modern technology to protect data, but also has to do with incentives. So if consent is required to create non-identifiable data, then organizations can just get consent for whatever analysis they want to do with the data. They don’t need to synthesize it anymore or create non-identifiable versions of it anymore. So by putting in place more steps to use data, we’re creating disincentives to use it in a privacy protective way. So if I have to get consent, I might as well get consent for whatever analysis and uses I was going to do with additional data. And I end up using identifiable information, which is less protective of the individual’s privacy rights. That’s one example of uncertainty and incentives.
Another one is around when you create non-identifiable data, privacy laws are binary in the sense that they treat data as identifiable or non-identifiable. And in practice, identifiability is on a spectrum. And so there are these thresholds that are used to determine when it moves from being personal data to non-personal data. And again, uncertainty around what are acceptable thresholds has made it difficult for organizations to know what they should do. So being more prescriptive or provide additional clarity on what are deemed to be acceptable thresholds would be very helpful because it reduces this uncertainty.
The more it’s clear what the rules are, it becomes easier for people to follow the rules. And when the rules are unknown, many organizations will do nothing because it’s the least risky option. So you’re creating disincentives for applying privacy-enhancing technologies. And that’s not ideal. I mean, ideally you want to incentivize organizations to apply the best available privacy-enhancing technologies to protect the privacy of individuals and then to enable them to use that data responsibly. I think reducing uncertainty and putting in place the right incentive or removing disincentives are two kind of big headlines that I would use to characterize a lot of the things that I think would be very helpful.
The other thing to keep in mind is… I think this is really important because in a lot of conversations, people talk about uses of data in terms of risk. There’s all these risks to using data, the risk of data being misused and so on. But there are also a lot of benefits to using data. We have to also keep in mind the benefits side of the equation that data uses can be very beneficial for society. And they can have tremendous economic benefits as well. For companies in Ontario, for Canadian organizations, I mean, we have to compete with the world. It’s not just about managing risk. It’s about managing risk, but also gaining the benefits of using these data sets.
PK:
Very interesting. Thank you again, so much, Khaled, for joining me on Info Matters. This is indeed a very complex topic and you’ve really helped us put it into context for our listeners. The possibilities of synthetic data do seem promising as a way of creating useful data sets for addressing urgent problems and delivering real-world results. For listeners who want to learn more about synthetic data, feel free to reach out to Khaled. And for those who want to learn more about de-identification techniques and other privacy-enhancing technologies, you can visit our website at ipc.on.ca. You can also contact our office for assistance and general information about Ontario’s access and privacy laws. We’ve come to the end of another episode of Info Matters. Thank you so much for listening, and until next time.
I’m Patricia Kosseim, Ontario’s Information and Privacy Commissioner, and this has been Info Matters. If you enjoyed the podcast, leave us a rating or review. If there’s an access or privacy topic you’d like us to explore on a future episode, we’d love to hear from you. Send us a tweet @IPCinfoprivacy, or email us at @email. Thanks for listening and please join again for more conversations about people, privacy, and access to information. If it matters to you, it matters to me.